to begin with,
What is Machine Learning?
It is a branch of computer science under Artificial Intelligence where the algorithms automatically improve by experience and the data given. The idea is that the system learns from the data provided and understands the data, finds patterns, and gives its predictions to make decisions.

Machine Learning lifecycle there are 3 major types of machine learning, namely Supervised learning, unsupervised learning, Reinforcement learning.
Supervised Learning:
Machine learning type where the model is trained with labeled data (categorized input and output data) to make the predictions. Linear regression, Decision tree, Support vector machines are some of the algorithms used for supervised learning.
Unsupervised Learning:
The type of machine learning where the model is provided unlabeled data to identify patterns and come to a conclusion. The example of algorithms are K-means clustering and Apriori.
Semi-supervised Learning:
This is nothing but the combination of supervised and unsupervised learning where the data is partially labeled and partially unlabeled. self-trained Naïve Bayes classifier is an example of semi-supervised learning algorithms.
Reinforcement Learning:
Algorithms that are self-interpreting but learns based on the rewards through the trial-and-error method.
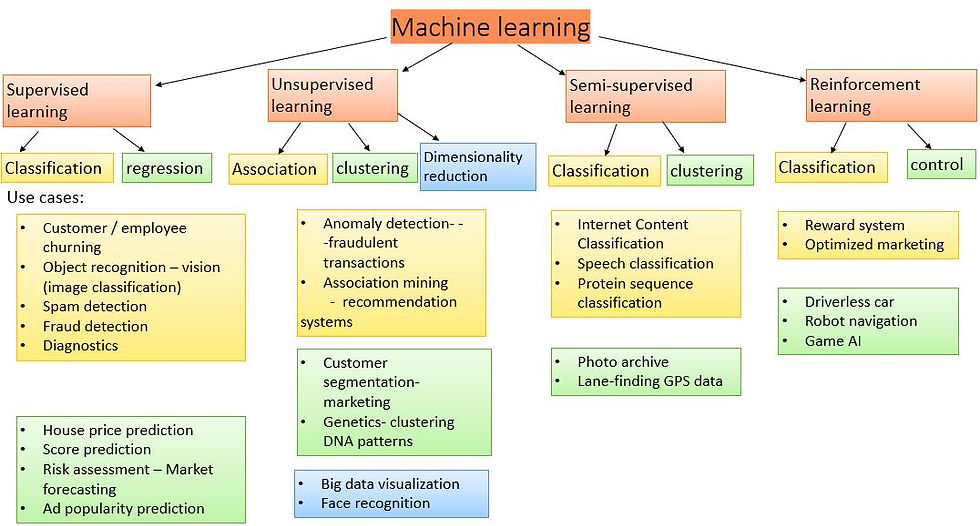
What are the steps, Machine learning lifecycle has?
Firstly, a Machine learning project has a data collection where the data from different sources and of different formats are collected, then comes the data preprocessing, here the data is prepared i.e., making the raw data suitable for modeling. The data preprocessing also includes data cleansing (handling missing data). The data is then analyzed to understand the data, features and find some hidden relations. Then comes the model building and training followed by model testing (we’ll dig into these steps in the later blogs with examples). Finally, the model is deployed. Oh, wait, it is not yet … as I mentioned in the beginning Machine learning models learn with experience, which means as the data increases the models are improved and maintained which is Monitoring.
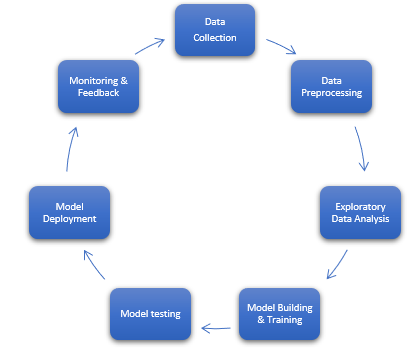
Today, Machine learning use cases are helpful for various business problems. Here are few examples of Machine Learning applications in different sectors that you might have not realized…

Comentários